The Role of AI in Litigation and Competition Expert Analysis: A Conversation with Anindya Ghose
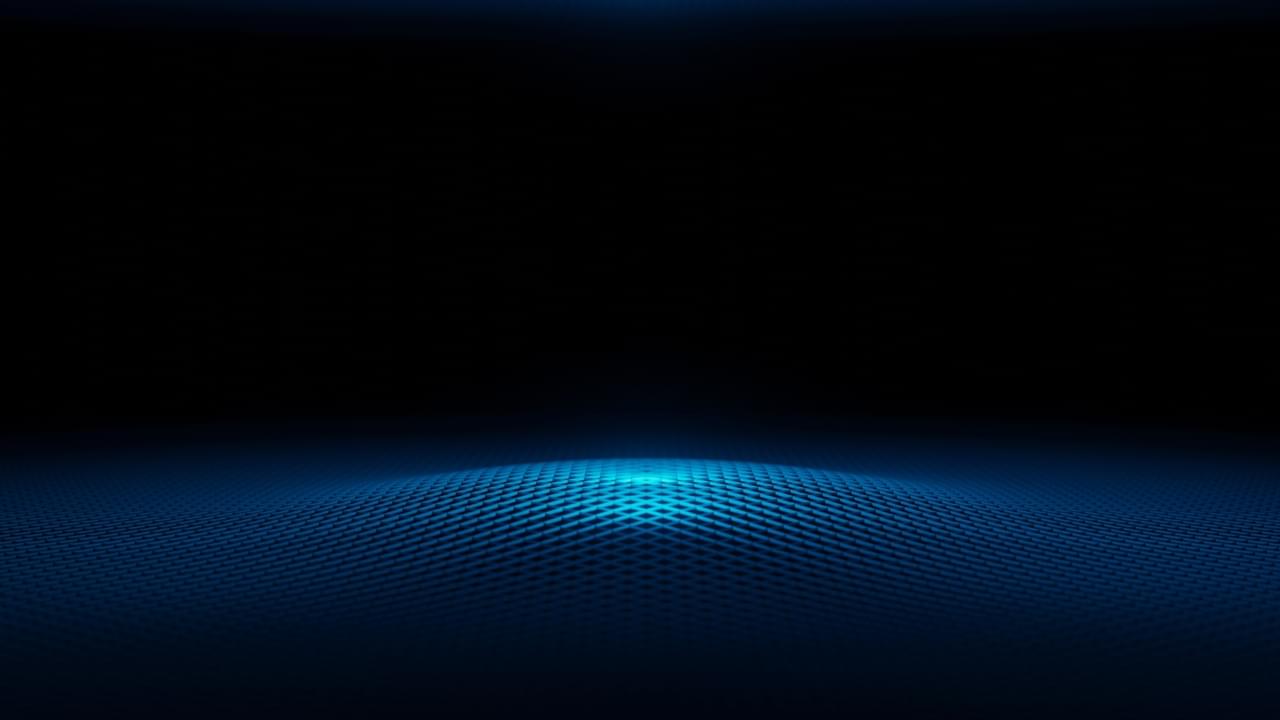
Share
As AI becomes increasingly pervasive in all aspects of life and the economy, its use in legal and expert witness analysis is on the rise.
In Ross v. United States, [1] the District of Columbia Court of Appeals acknowledged AI’s novel role in generating opinions. In noting the Court’s “appropriate” utilization of AI, an Associate Judge on the case issued a concurring opinion:
It strikes me that the thoughtful use employed by both of my colleagues are good examples of judicial AI tool use for many reasons—including the consideration of the relative value of the results—but especially because it is clear that this was no delegation of decision-making, but instead the use of a tool to aid the judicial mind in carefully considering the problems of the case more deeply.
AI has also been used in expert analysis for litigation between parties, sometimes based on appropriate methods and sometimes not. For example, experts have used AI tools to process or classify unstructured information, including social media posts, consumer-generated reviews of products, or online consumer forum posts, to arrive at highly structured data that are then ripe for further analysis and drawing relevant inferences. In Turtle Island Foods v. Kevin Stitt, et al., expert witnesses specializing in consumer behavior used a natural language processing tool to identify associations between free-text responses to a survey they conducted to gather consumer views about plant-based products.[2]
AI has also been used in expert sentiment analysis, which involves identifying the emotional tone of words. In The Cookie Department, Inc., v. Hershey Company, et al., an expert witness performed sentiment analysis on a selection of the Hershey Company’s consumer product reviews using a machine learning AI model.[3]
In this Q&A, Compass Lexecon experts Niall MacMenamin, Vendela Fehrm, and Zhaoning (Nancy) Wang, speak with Professor Anindya Ghose from NYU Stern about his academic research in AI and how AI can be applied in litigation. Professor Ghose has extensive expertise in evaluating and applying large language models (LLMs), which are starting to be applied in economic litigation projects. Compass Lexecon experts have worked closely with Professor Ghose to evaluate, develop, and maintain LLMs for use in litigation. Through this conversation, we demonstrate that there is a role for AI in expert analysis when the appropriate oversight is applied.
Before we talk about your use of AI in litigation, it would be useful to know more about your research that has involved AI. Can you briefly describe what you have studied and what you have learned?
In my academic research, I have studied AI in several different contexts:
- First, using Generative AI tools, I have looked at how AI performs compared to humans in certain business tasks such as the creation of digital advertising.
- Second, I have looked at how the introduction of AI in an industry affects individuals’ decisions to create content and their productivity.
- Third, I have used advanced AI techniques to analyze and categorize large volumes of different kinds of data using machine learning, sentiment analyses, and deep learning. My recent book, Thrive: Maximizing Well-Being in the Age of AI, ties much of my research together.[4] In Thrive, my co-author, Ravi Bapna, and I study how AI is positively influencing the aspects of our daily lives that we care about most: our health and wellness, relationships, education, the workplace, and domestic life. We also provide a time-tested multi-method framework that businesses can adopt to navigate their AI transformation journeys.
Can you provide examples of you have studied AI within those three contexts?
On the topic of how AI performs compared to humans in certain business tasks, my co-authors and I studied the use of AI in digital advertising effectiveness. This is a topic that I have spent a considerable portion of my career analyzing, because these technologies not only affect the tech industry, with companies like Meta, Google, Amazon, Pinterest, TikTok, X, and Snapchat providing platforms for advertisers, it also affects publishers, news outlets, advertisers, and users of those platforms, which includes nearly everyone. In “The Impact of Generative AI on Advertising Effectiveness,” my co-authors—Hyesoo Lee, Panagiotis Adamopoulos, and Vilma Georgia Todri,—and I were interested in knowing, within a digital advertising setting, whether AI outperforms humans at some of the important advertising functions, like increasing the probability of sale.[5]
Our findings suggest that generative AI-“modified” display ads, in which the visual aspect of a display ad was modified by AI, do not outperform human experts ads, but generative AI-“created” display ads, in which the visual aspect of a display ad was created from scratch by AI, do. We argue that this indicates the proficiency of visual generative AI in creation tasks, but its limitations in modification tasks.
Figure 1: Examples of Ads Created by Human Expert, Modified by GenAI, and Created by GenAI[6]
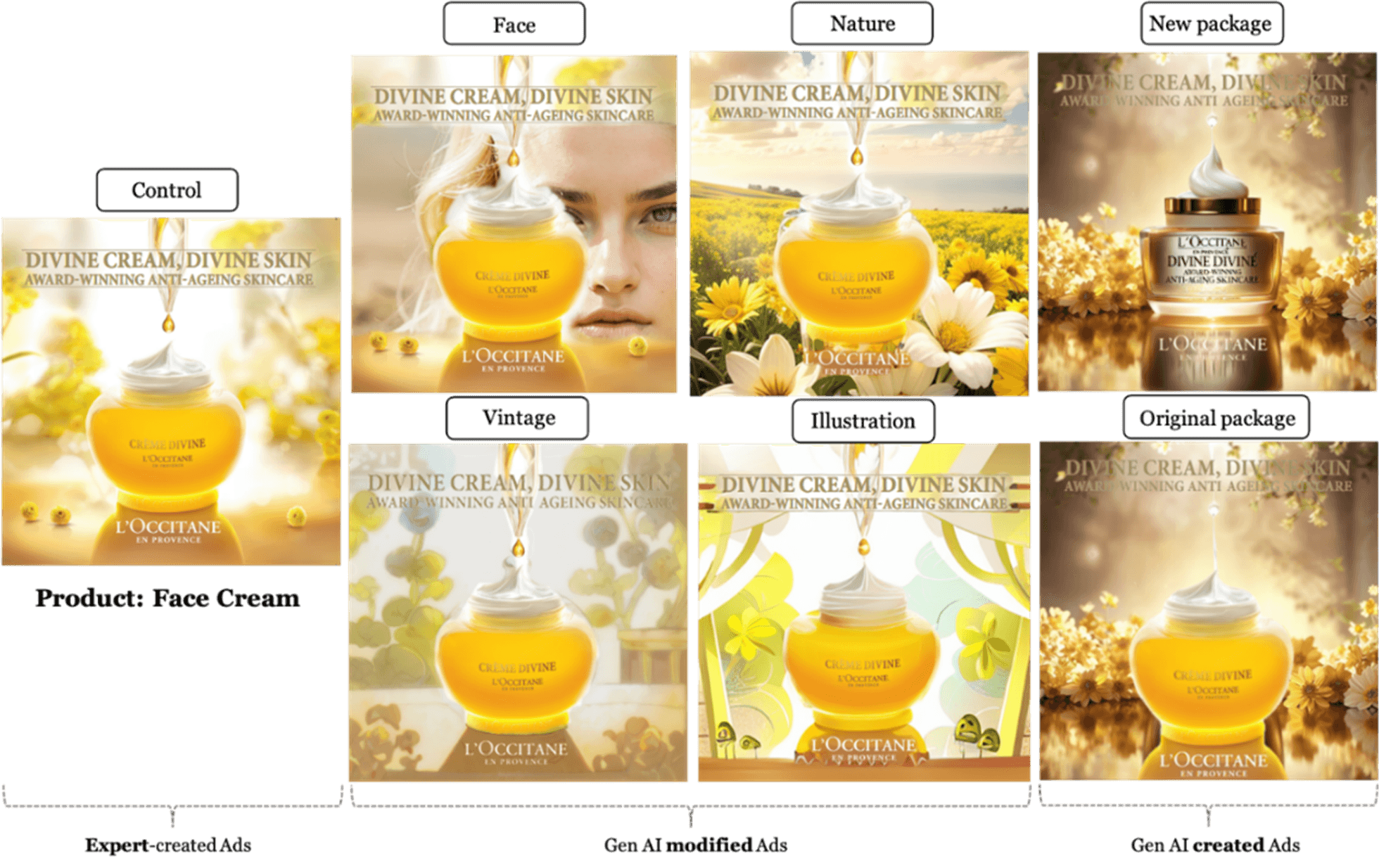
In “Generative AI and Content Creators: Evidence from Digital Art Platforms,” my co-authors Hongxian Huan, Runshan Fu, and I were interested in learning about how AI can affect people’s decisions to make content and how it impacts their productivity.[7] To that end, we studied how AI affects digital art creators’ activities on digital platforms. We found that the introduction of AI to a platform led to a significant decrease in its content creators’ activities on the platform, but the effect varied across creators. Conversely, prohibiting AI on a platform has a more nuanced effect on decisions to create content and productivity; some creators left the platform, while others increased their activities on the platform. The findings from this paper are relevant to several of the copyright infringement lawsuits that are currently ongoing as they speak to the incentives of content creators in the face of the adoption of AI by digital platforms.
I have also used AI as a tool in my research, primarily to analyze and categorize data using “Transformer” and “BERT”-like models that are at the heart of LLMs like ChatGPT. In “Gendered Information in Resumes and Hiring Bias: A Predictive Modeling Approach,” my co-authors, Prasanna Parasurama, João Sedoc, and I studied whether male and females write their resumes differently and how those differences affect hiring outcomes.[8] We used AI to quantify the extent of gendered information in resumes and then predicted the effect of gendered information on call-back decisions. Our AI model was trained on a large sample of hundreds of thousands of resumes to quantify gendered information and inductively learn the differences between male and female resumes. We found that gendered information plays a role in hiring bias—women who exhibit masculine characteristics in their resumes are less likely to receive a callback after controlling for job-relevant characteristics.
The use of AI in my work in litigation draws from all three of these areas, but most significantly from the third category, which involves applying AI as a tool for data analysis and categorization. For example, I utilized a sentiment analysis tool to analyze social media posts.
How can AI be utilized in litigation?
AI has become an increasingly popular tool in litigation, particularly in competition law cases.[9] AI-driven methods, including machine learning and deep learning, allow for the processing of large volumes of structured and unstructured data, making it possible to extract meaningful insights at a speed and scale unattainable by traditional methods. AI can also enhance consistency by applying standardized analysis across multiple documents without variation introduced by human interpretation. These capabilities of AI are particularly relevant when working with user-generated content from social media or consumer reviews about products. These data, once categorized in a systematic way using AI-enabled techniques, can form the basis of powerful economic analyses.
Notably, this categorization process is not left solely to AI—indeed, human experts must oversee and refine the process to ensure accuracy and replicability, thereby reinforcing the reliability of AI-driven insights.
One of the first steps when using an AI-based approach is to select the right LLM for the project. General-purpose LLMs, such as GPT-4, Claude, Perplexity, BERT, Llama, Grok, and Mistral, offer broad adaptability but may not always be the most suitable tool for specific tasks. Specialized LLMs, on the other hand, are typically trained and optimized for particular purposes. For example, there are LLMs for legal work (e.g., CaselawGPT), academic research (e.g., SciBERT), analysis of social media and consumer data (e.g., Iris), and computer code generation (e.g., Codex).
LLMs have been used by expert witnesses in litigation matters to conduct “sentiment analysis,” which is a method of examining positive, negative, or neutral public reactions to statements made by individuals in media outlets and on platforms like X. If conducted correctly, sentiment analysis can provide insights into connections between statements and public perceptions, which can also inform further economic assessments about liability or damages that are central to the case. More broadly speaking, AI can also be used to evaluate economic models, predict market dynamics, and detect potential anticompetitive behavior.[10] For instance, AI-driven algorithms can facilitate price collusion and anticompetitive behavior in e-commerce marketplaces or other equivalent markets. AI-powered tools, especially LLMs, can be tailored for use in a litigation setting and also help streamline tasks such as reviewing confidential documents, identifying key patterns in regulatory filings, and conducting market analyses for merger cases.
What are some considerations and risks that someone should be aware of when using AI in litigation?
If you are considering using AI in litigation or elsewhere, you need to be aware of inherent risks in AI and ways to minimize or properly address them. One of the primary concerns is “model hallucination,” where AI generates false or misleading information that appears credible. Another concern is that most LLM models are not self-aware of hallucinations or other errors and fail to notify users of certainty in responses to prompts. This is particularly problematic in legal contexts, where factual accuracy is paramount. A recent study by Stanford RegLab and the Institute for Human-Centered AI found that LLMs provide output that is inconsistent with facts for between 58% and 88% of specific legal queries.[11]
Notably, the risk of hallucination in AI models has decreased over time due to advances in AI architecture, training techniques that incorporate human feedback in the fine-tuning process (reinforcement learning from human feedback (RLHF)) to refine their outputs based on real-world corrections, and retrieval-based methods that pull real-time data from external sources (e.g., legal databases, case law, financial records) before generating responses (retrieval-augmented generation (RAG)). These advances have improved factual accuracy and reduced hallucinations. Methods that can be used to identify false information include cross-checking results with reliable sources, checking for logical consistency, and spot-checking AI results based on human coding in a data sample.
LLMs that are guided by “few-shot examples” (i.e., a few examples that are provided as part of the prompt to guide or “train” the LLM on what the expected output would be) can be highly sensitive to the choice of those examples. Even a small variation in how a request is phrased can lead to significantly different outputs, raising concerns about reliability and bias. Methods that can be used to determine the sensitivity of the results to the selection of the examples include changing the examples and verifying the stability of the results, or using different numbers and types of examples.
Another risk for consideration is the confidentiality and privacy of data and information that is imported by users into an AI model. Some AI models do use user data for training and other purposes, while others do not by default use user data.[12] AI models also have user settings, which can be adjusted to ensure the data are handled confidentially.
In short, while AI can enhance efficiency and provide valuable insights, the use of AI in litigation is only effective when combined with appropriate human expert supervision. In particular, ensuring the accuracy and relevance of AI-generated outputs is crucial in a litigation context and requires careful model selection, validation, and oversight. This can be achieved by using a dedicated data science team with expertise in model development, maintenance, and implementation to optimize AI tools for economic analysis in litigation work. As AI continues to evolve, its role in litigation will depend not only on technological advancement but also on experts’ ability to apply it in a rigorous and defensible manner within the legal framework.
What other topics in AI do you expect to appear in litigation matters?
I can think of at least two areas. First, there has been a flurry of legal cases involving “fair use” vs. copyright infringement cases involving LLMs that have trained their algorithms using content from various digital platforms (e.g., various litigations involving OpenAI, including The Intercept Media, Inc. v. OpenAI, Inc., In re OpenAI ChatGPT Litigation, and others, including Kadrey v. Meta Platforms, Inc.). Assessing and quantifying potential damages on such matters will require expertise in AI because traditional damages methodologies may not be sufficient. Second, I can imagine issues related to vertical integration, foreclosure, exclusive dealing, and tying/bundling that may emerge in the rapidly changing AI tech stack that consists of semiconductor chips, cloud computing, data centers, LLMs, and AI applications. If a single company builds a dominating presence in each layer of the AI tech stack, I presume that will prompt the Federal Trade Commission and Department of Justice to look into these issues in the near future. The FTC recently launched a probe related to this.[13]
Finally, concerns with privacy issues of data used for AI model training, false advertising of products that involve AI-generated info, and the ownership of AI-generated content will continue to arise. I suspect there will also be DOJ and FTC probes around these issues as the technology and markets evolve.
References
-
Ross v. United States, No. 23-CM-1067, 2025 WL 561432 (D.C. February 20, 2025).
-
Expert Report and Affidavit of Professor Adam Feltz, Ph.D. and Professor Silke Feltz, Ph.D., Turtle Island Foods and Plant Based Foods Association, v. Kevin Stitt, in his official capacity as Oklahoma Governor, and Blayne Arthur, in her official capacity as Oklahoma Commissioner of Agriculture, May 30, 2023.
-
Expert Report and Affidavit of Amanda Schlumpf, The Cookie Department, Inc., v. The Hershey Company, et al., May 31, 2022, United States District Court, N.D. California.
-
Bapna, Ravi, and Anindya Ghose, Thrive: Maximizing Well-Being in the Age of AI, The MIT Press, 2024.
-
Lee, Hyesoo, Panagiotis Adamopoulos, Vilma Georgia Todri, and Anindya Ghose, “The Impact of Generative AI on Advertising Effectiveness,” ICIS 2024 Proceedings, 12, 2024.
-
Lee, Hyesoo, Panagiotis Adamopoulos, Vilma Georgia Todri, and Anindya Ghose, “The Impact of Generative AI on Advertising Effectiveness,” ICIS 2024 Proceedings, 12, 2024, Figure 1.
-
Huang, Hongxian, Runshan Fu, and Anindya Ghose, “Generative AI and Content Creators: Evidence from Digital Art Platforms,” Working Paper, May 21, 2024, available at http://dx.doi.org/10.2139/ssrn.4670714.
-
Parasurama, Prasanna, João Sedoc, and Anindya Ghose, “Gendered Information in Resumes and Hiring Bias: A Predictive Modeling Approach,” Working Paper, April 4, 2022, available at http://dx.doi.org/10.2139/ssrn.4074976.
-
See, e.g., Hofmann, Herwig C.H., and Isabella Lorenzoni, “Future Challenges for Automation in Competition Law Enforcement,” Stanford Computational Antitrust, 2023, Vol. III, pp. 36–54 at pp. 37–38 (“In competition law enforcement, for instance, competition authorities have started to […] invest in AI as a strategy to better understand and monitor the current dynamics in markets and the AI tools used by market participants to decide real-time pricing strategies and supply chain flows.”); Coglianese, Cary, “AI For the Antitrust Regulator,” ProMarket, June 6, 2023, available at https://www.promarket.org/2023/06/06/ai-for-the-antitrust-regulator/; “How AI is transforming the legal profession (2025),” Thomson Reuters, January 16, 2025,available at https://legal.thomsonreuters.com/blog/how-ai-is-transforming-the-legal-profession/.
-
See, e.g., Korinek, Anton, “Generative AI for Economic Research: Use Cases and Implications for Economists,” Journal of Economic Literature , Vol. 61, No. 4, 2023, pp. 1281–1317; Coglianese, Cary, and Alicia Lai, “Antitrust by Algorithm,” Stanford Computational Antitrust, Vol. II, 2022, pp. 1–22; Francisco Beneke, and Mark-Oliver Mackenrodt, “Artificial Intelligence and Collusion,” International Review of Intellectual Property and Competition Law, Vol. 50, 2019, pp. 109–134.
-
Dahl, Matthew, Varun Magesh, Mirac Suzgun, and Daniel E. Ho, “Large Legal Fictions: Profiling Legal Hallucinations in Large Language Models,” Journal of Legal Analysis, Vol. 16, No. 1, 2024, pp. 64–93.
-
This information is provided in the Terms of Use for each model.
-
“Trump’s FTC advances broad antitrust probe of Microsoft, Bloomberg News reports,” Reuters, March 12, 2025, available at https://www.reuters.com/technology/trumps-ftc-moves-ahead-with-broad-antitrust-probe-microsoft-bloomberg-news-2025-03-12/.